Quantum noise, qubit decoherence, and quantum control inaccuracies cause errors in quantum computations.
We are excited to announce we have detailed a new breakthrough in the ability to significantly reduce these errors in a preprint now on arXiv.org. Using this new error mitigation strategy, which we call debiasing via frugal symmetrization, or just debiasing for short, our customers will benefit from the computing power of increased qubit volume without being undercut by inaccurate computations.
What is debiasing?
The influence of environmental interference on the control of the atoms used in quantum computing means qubits do not always perform perfectly in real-world computation. Even slight imperfections affect the results of quantum algorithms. Error mitigation strategies, such as debiasing, attempt to correct the impact of these imperfections.
This problem has always been considered a major roadblock to building useful quantum computers at scale. This is because the more qubits you have, the larger the opportunity for deviation between the ideal outcome of your algorithm and your actual outcome becomes, meaning a drop in the accuracy of your quantum computation. This phenomenon impairs a quantum computer’s ability to solve real-world problems.
In our error mitigation strategy, we use computational symmetries of quantum algorithms – qubit assignment or gate decomposition, for example – to diversify the effect of inaccuracies across multiple implementations. When the outcomes of different implementations are then combined, the diversified inaccuracies cancel out resulting in a more accurate outcome. Since every quantum circuit already needs to be executed multiple times to gather enough statistics, we can simply vary the implementation of those executions to achieve error mitigation with no overhead in execution time.
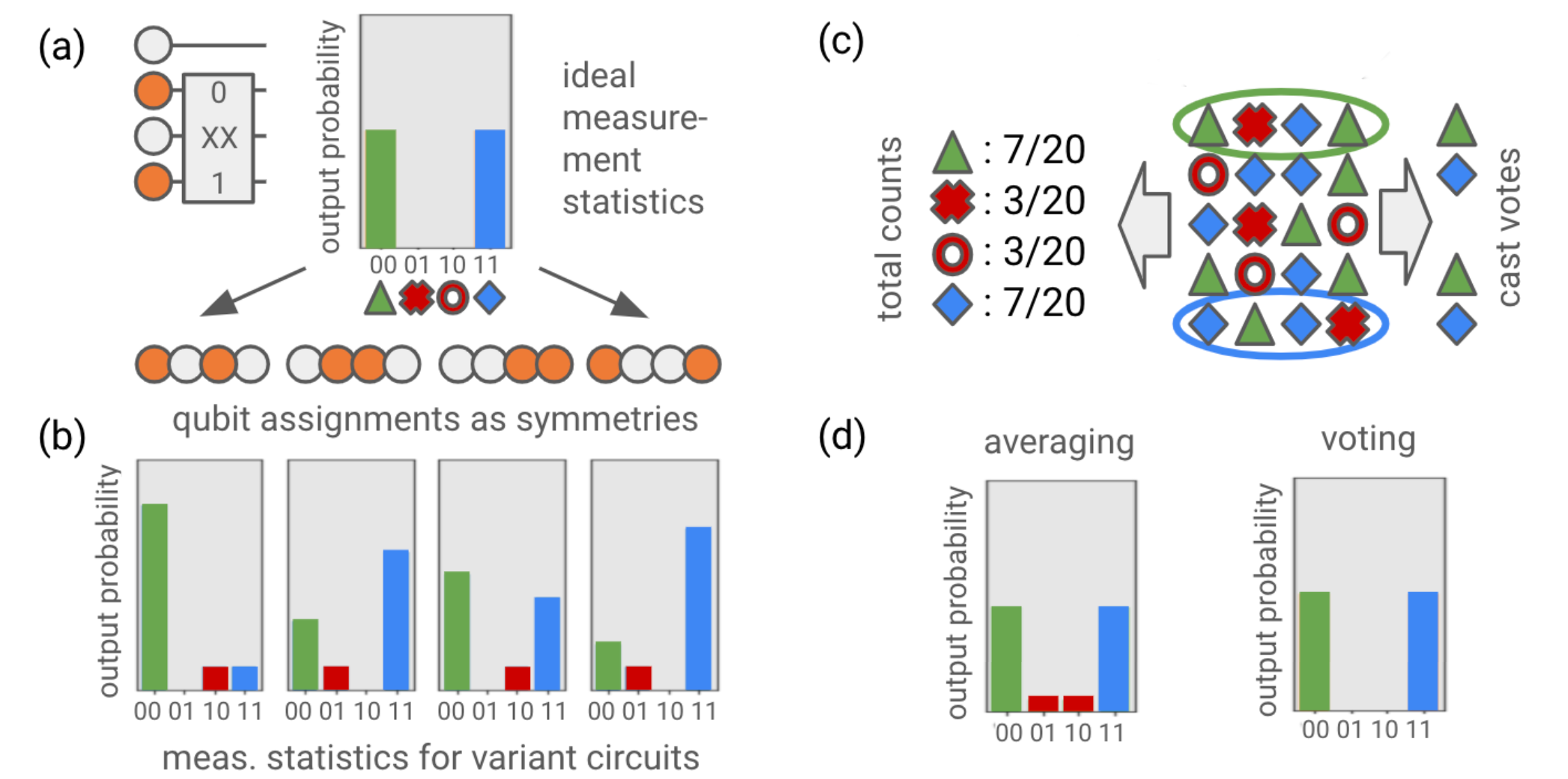
The potential of debiasing
Measurement statistics of multiple implementations of a quantum computation can be aggregated in different ways that would benefit the accuracy of the aggregated results depending on the algorithm. The more we know about the output distribution of a particular algorithm, the more we can exploit its symmetries to distill original statistics from multiple implementations with inaccuracies.
For example, for Quantum Machine Learning or Chemistry problems, where the output probability distribution can vary significantly between the circuits of the same type, one could use aggregation by averaging, the default behavior for debiasing. However, for phase and amplitude estimation algorithms, where the answer is encoded in a set of equally probable states, a more specialized nonlinear aggregation strategy, which we call sharpening, can lead to much larger performance gains. Developing more adaptive or application-specific debiasing and aggregation methods can unlock significant performance improvement for NISQ-era quantum computers.
We are excited to see what gains debiasing will unlock for our customers as we continue to scale our quantum systems! The paper was authored by IonQ’s Andrii Maksymov, Jason Nguyen, Yunseong Nam and Igor Markov, and we congratulate them for their work. Yunseong is also a member of the University of Maryland’s Department of Physics, which continues to be a close partner for our research efforts.